How accurately does anyone know the global distribution of income?
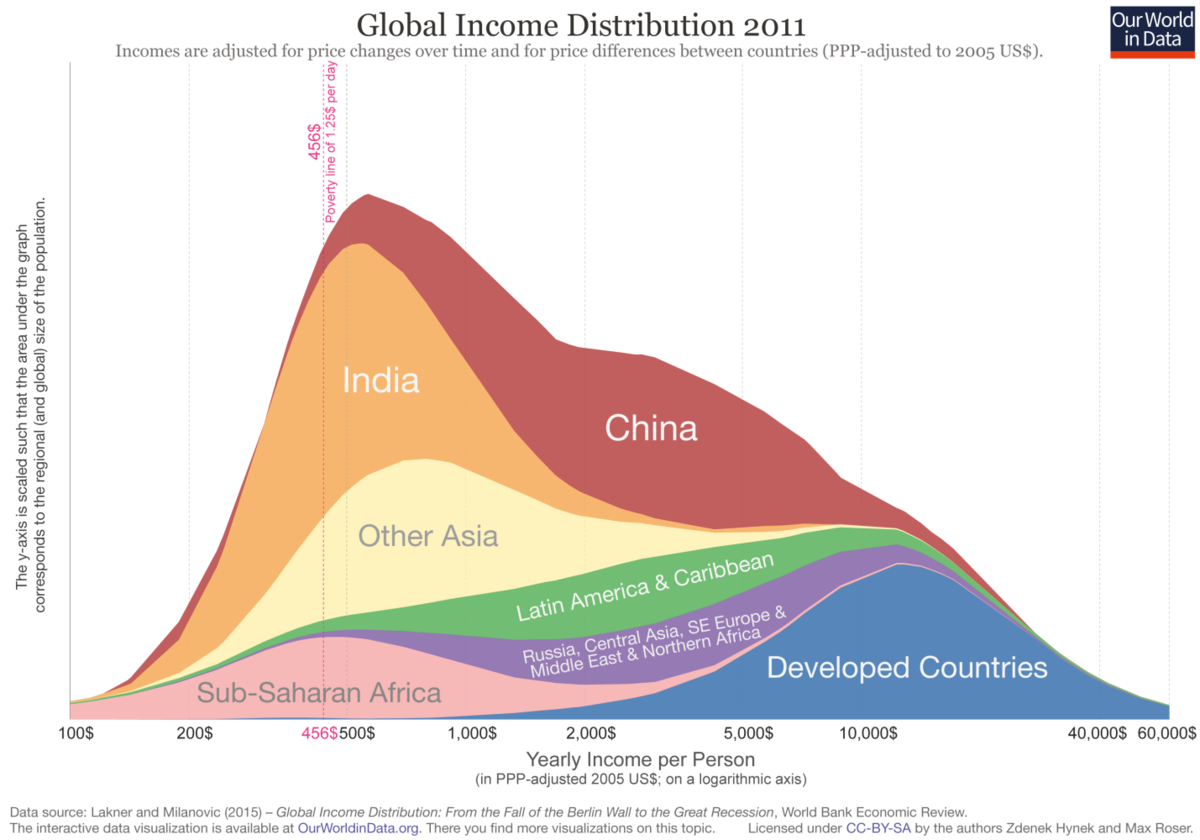
People in the effective altruism community often refer to the global income distribution to make various points:
- The richest people in the world are many times richer than the poor.
- People earning professional salaries in countries like the US are usually in the top 5% of global earnings and fairly often in the top 1%. This gives them a disproportionate ability to improve the world.
- Many people in the world live in serious absolute poverty, surviving on as little as one hundredth the income of the upper-middle class in the US.
Measuring the global income distribution is very difficult and experts who attempt to do so end up with different results. However, these core points are supported by every attempt to measure the global income distribution that we’ve seen so far.
The rest of this post will discuss the global income distribution data we’ve referred to, the uncertainty inherent in that data, and why we believe our bottom lines hold up anyway.
Will MacAskill had a striking illustration of global individual income distribution in his book Doing Good Better, that has ended up in many other articles online, including our own career guide:
The data in this graph was put together back in 2012 using an approach suggested by Branko Milanovic, at the time lead economist in the World Bank’s research department, and author of The Haves and the Have-Nots. Incidentally, Milanovic went on to achieve mainstream fame for the so-called ‘elephant graph’. For the bottom 80% of the income distribution we used World Bank figures from their database ‘PovcalNet’. As this data set was not considered reliable for the top 20% of the income distribution, we substituted them with figures from Branko Milanovic’s own work compiling national household surveys.
Some have questioned whether the graph gives a misleading picture of global inequality. How seriously should we take it?
One obvious concern is that this distribution is based on income surveys from 2008, and the global income distribution may have changed since then. Strong economic growth in countries like China has improved the lot of people in the middle of the income distribution, while the Great Recession in 2008-10 suppressed incomes in rich countries more than poor countries. Hellebrandt and Mauro attempts to estimate how the income distribution changed between 2003 and 2013, and finds a quite significant shift.1
Why are we still using numbers from 9 years ago? Complete and consistent global income distribution estimates arrive infrequently and at a substantial delay, because they rely on surveys trickling in from over 150 countries around the world, and being made comparable. 2008 is still the last year for which we are aware of publicly available and compatible survey figures across the whole distribution. We are working to get access to newer figures that are not yet public, though they will only bring us up to 2011.
But that’s only the beginning of the difficulties. There are lots of ways different organisations might produce different numbers:
- The underlying survey data may be inaccurate or sample differently. For example, different polling groups will have different ways of trying to question a representative cross-section of people in a country about their income. Needless to say this gets very challenging. Imagine trying to make sure you’ve fairly sampled the poorest 20% of people in India, or the Democratic Republic of Congo. The people you want to sample may be in rural areas without access to any telecommunications. How do you know you’ve got the right number of people from these groups in your measurements? And how do you measure the equivalent income of people who grow food for their own consumption, rather than receive a salary? PovcalNet is up-front about the serious challenges they face:
More than 2 million randomly sampled households were interviewed in these surveys, representing 96 percent of the population of developing countries. Not all these surveys are comparable in design and sampling methods. Non representative surveys, though useful for some purposes, are excluded from the calculation of international poverty rates. … No data are ideal. International comparisons of poverty estimates entail both conceptual and practical problems that should be understood by users.
In line with its desire to measure the extent of effective poverty around the world, PovcalNet uses measures of consumption where they are available. This means that income people receive which they then save is not counted, while spending funded by past savings is counted, and savings this year will appear in future years’ consumption. Our World In Data has more information on how this is done.
If you’d like to learn more about how questionable data coming out of the developing world can be, a good source would be Poor Numbers by Morten Jerven.
Getting enough data about the top 1% of earners is difficult in a different way: they represent only a small fraction of respondents in surveys, their income sources are more varied, and their incomes differ enormously.
Different ways of adjusting for ‘purchasing power’. Money goes further in poorer countries, and any sensible attempt to look at global income will account for this. But how do you compare the value of two currencies when the people in the relevant countries are buying very different things? Very few identical products are bought in both rural Kenya and Switzerland, so any attempt to compare the practical purchasing power of Swiss francs and Kenyan shillings is going to be imprecise. Moreover, even within countries people at different parts of the income distribution consume very different bundles of goods, and therefore are affected by different prices. Economists do their best to sample what people are buying in a range of places, how similar their quality is to goods elsewhere, and what they cost – but only so much is possible. In one dramatic case, a revision of purchasing power parity weights by the World Bank in 2005 cut China’s purchasing power parity-adjusted GDP by 40 per cent. Then in 2014 it was revised back up based on surveys conducted in 2011, suddenly making it the largest economy in the world
(maybe, anyway).Finally, how do people deal with the varied cost of living in different places within a country? I’ve never seen these adjustments made. And it’s unclear how much they should be made. One way people choose to spend their income to improve their lives is to live in expensive cities!
Different ways of dealing with household size. Sometimes income data is given ‘per capita’, and other times it’s given ‘per household’. This can change the shape of an income distribution, because globally larger families have lower incomes.
Larger families also have greater ‘economies of scale’ (e.g. they might share a single house or car). When economists want to take household income from surveys and ‘individualise’ the figures to compare across households of different sizes, they use ‘equivalence scales’. But estimates of the right equivalence scales differ a remarkable amount. Using one method, a couple and one child living on $20,000 collectively, have an effective individual income of $15,200. Using a method at the other extreme, the figure is $9,100. Alternatively, you might just divide total household income by the number of family members and ignore any of the effects of family structure (this is the approach taken in PovcalNet and Milanović’s figures). This creates another source of variation in how the survey data is processed before you see it.
Different dollar units. Figures for global income comparisons are usually given in ‘international dollars’. Occasionally 1990 international dollars are used for comparison of changes in data over long time periods. Other times you’ll find figures in 2000 international dollars, 2011 international dollars, or whatever year the data were released. Inflation between these different time periods can move the figures by 10-40%.
Are the figures after tax or pre-tax? Gallup Polling and Hellebrandt and Mauro (2015) report income pre-tax. The Brankovic figures used above are post-tax. PovcalNet doesn’t say on their site, but in correspondence I’ve been told “in principle the figures are post-tax” (the World Bank is forced to draw on many varied data sources). This alone could create a 25-50% gap between them.
Is pre-tax or post-tax the better way to do things? Reasonable people can disagree about this. People in poorer countries pay less tax, which in a sense boosts their spending power. But they also get fewer services from their governments in return, forcing them to pay for them out of pocket. On the other hand, if high income earners in a given country are funding financial transfers to people on lower incomes – rather than services they personally receive – it makes more sense to exclude those taxes from their effective income.
One person sent us figures from Gallup Polling that seemed to dramatically conflict with our graph – a median income of $9,733 vs the $1,272 we pulled out of the World Bank’s PovcalNet. The first big adjustment is that the $10,000 figure is for households, whereas the chart we use gives figures for individuals. The per person income figure from Gallup is the more modest $2,920.
If that person had looked around, they would have found that other sources give different numbers again. For example, Hellebrandt and Mauro, which I mentioned above, offers a global median income of $2,010 in 2013.2 Milanovic’s estimate was $1,225 for 2005, and $1,480 for 2008.3
A substantial fraction of those differences can be explained by rising incomes over time, and the fact that the two higher numbers are pre-tax, and the lower two post-tax. What explains the remainder? The information required to figure that out isn’t publicly available, and answering that question is really a job for an expert in the field rather than a dilettante such as myself. One possibility is that the surveys used by the World Bank go further into poor, dangerous and rural communities than those by Gallup (a private polling company). Evidence of this is that in their income tables Gallup appears to only have surveyed the capital city of the Democratic Republic of Congo. In addition, as far as I could see Gallup’s polling data has not yet been published in an economics journal, so there could be quite a few methodological differences with the rest of the literature.
All that said, given the range of choices researchers are required to make, a difference of this size isn’t much of a surprise.
Political scientist Merle Kling once proposed three ‘iron laws of social science’, and they apply here as much as anywhere:
- Sometimes it’s this way, and sometimes it’s that way.
- The data are insufficient.
- The methodology is flawed.
These figures are approximations. However, having had personal experience with social science data, the rigour here is better than I would have expected going in. As far as I can tell most researchers are making defensible decisions while trying to produce these estimates.
And despite the challenges, these bottom lines remain true in every estimate of the global income distribution I’ve seen so far:
- The richest people in the world are many times richer than the poor.
- People earning professional salaries in countries like the US are usually in the top 5% of global earnings and sometimes in the top 1%. This gives them a disproportionate ability to improve the world.
- Many people in the world live in serious absolute poverty, surviving on as little as one hundredth the income of the upper-middle class in the US.
Notes and references
- Hellebrandt, Tomas and Mauro, Paolo (2015) – The Future of Worldwide Income Distribution (April 1, 2015). Peterson Institute for International Economics Working Paper No. 15-7. Available at SSRN or http://dx.doi.org/10.2139/ssrn.2593894.↩
- Incidentally, it’s unlikely they could have had global survey data compiled for 2013 by 2015, as individual country distributions for 2013 are only becoming available now. So they probably used modelling assumptions about growth at different parts of the distribution. The more you know!↩
- The former of these is in The Haves and the Have-Nots vignette 3.2. The latter figure is from personal correspondence.↩