#162 – Mustafa Suleyman on getting Washington and Silicon Valley to tame AI
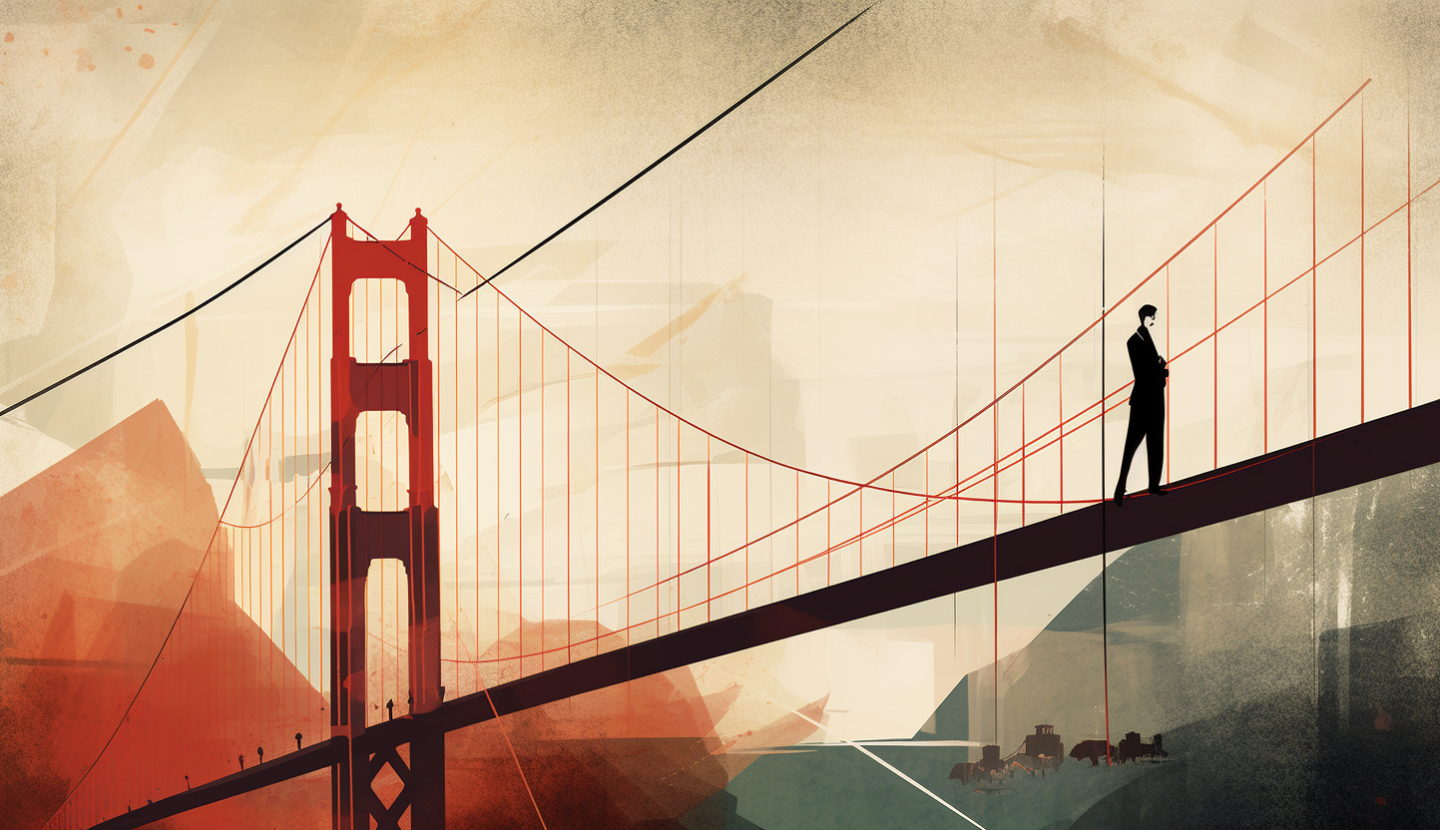
Mustafa Suleyman was part of the trio that founded DeepMind, and his new AI project is building one of the world’s largest supercomputers to train a large language model on 10–100x the compute used to train ChatGPT.
But far from the stereotype of the incorrigibly optimistic tech founder, Mustafa is deeply worried about the future, for reasons he lays out in his new book The Coming Wave: Technology, Power, and the 21st Century’s Greatest Dilemma (coauthored with Michael Bhaskar). The future could be really good, but only if we grab the bull by the horns and solve the new problems technology is throwing at us.
On Mustafa’s telling, AI and biotechnology will soon be a huge aid to criminals and terrorists, empowering small groups to cause harm on previously unimaginable scales. Democratic countries have learned to walk a ‘narrow path’ between chaos on the one hand and authoritarianism on the other, avoiding the downsides that come from both extreme openness and extreme closure. AI could easily destabilise that present equilibrium, throwing us off dangerously in either direction. And ultimately, within our lifetimes humans may not need to work to live any more — or indeed, even have the option to do so.
And those are just three of the challenges confronting us. In Mustafa’s view, ‘misaligned’ AI that goes rogue and pursues its own agenda won’t be an issue for the next few years, and it isn’t a problem for the current style of large language models. But he thinks that at some point — in eight, ten, or twelve years — it will become an entirely legitimate concern, and says that we need to be planning ahead.
In The Coming Wave, Mustafa lays out a 10-part agenda for ‘containment’ — that is to say, for limiting the negative and unforeseen consequences of emerging technologies:
- Developing an Apollo programme for technical AI safety
- Instituting capability audits for AI models
- Buying time by exploiting hardware choke points
- Getting critics involved in directly engineering AI models
- Getting AI labs to be guided by motives other than profit
- Radically increasing governments’ understanding of AI and their capabilities to sensibly regulate it
- Creating international treaties to prevent proliferation of the most dangerous AI capabilities
- Building a self-critical culture in AI labs of openly accepting when the status quo isn’t working
- Creating a mass public movement that understands AI and can demand the necessary controls
- Not relying too much on delay, but instead seeking to move into a new somewhat-stable equilibria
As Mustafa put it, “AI is a technology with almost every use case imaginable” and that will demand that, in time, we rethink everything.
Rob and Mustafa discuss the above, as well as:
- Whether we should be open sourcing AI models
- Whether Mustafa’s policy views are consistent with his timelines for transformative AI
- How people with very different views on these issues get along at AI labs
- The failed efforts (so far) to get a wider range of people involved in these decisions
- Whether it’s dangerous for Mustafa’s new company to be training far larger models than GPT-4
- Whether we’ll be blown away by AI progress over the next year
- What mandatory regulations government should be imposing on AI labs right now
- Appropriate priorities for the UK’s upcoming AI safety summit
Get this episode by subscribing to our podcast on the world’s most pressing problems and how to solve them: type ‘80,000 Hours’ into your podcasting app. Or read the transcript.
Producer and editor: Keiran Harris
Audio Engineering Lead: Ben Cordell
Technical editing: Milo McGuire
Transcriptions: Katy Moore