#185 – Lewis Bollard on the 7 most promising ways to end factory farming, and whether AI is going to be good or bad for animals
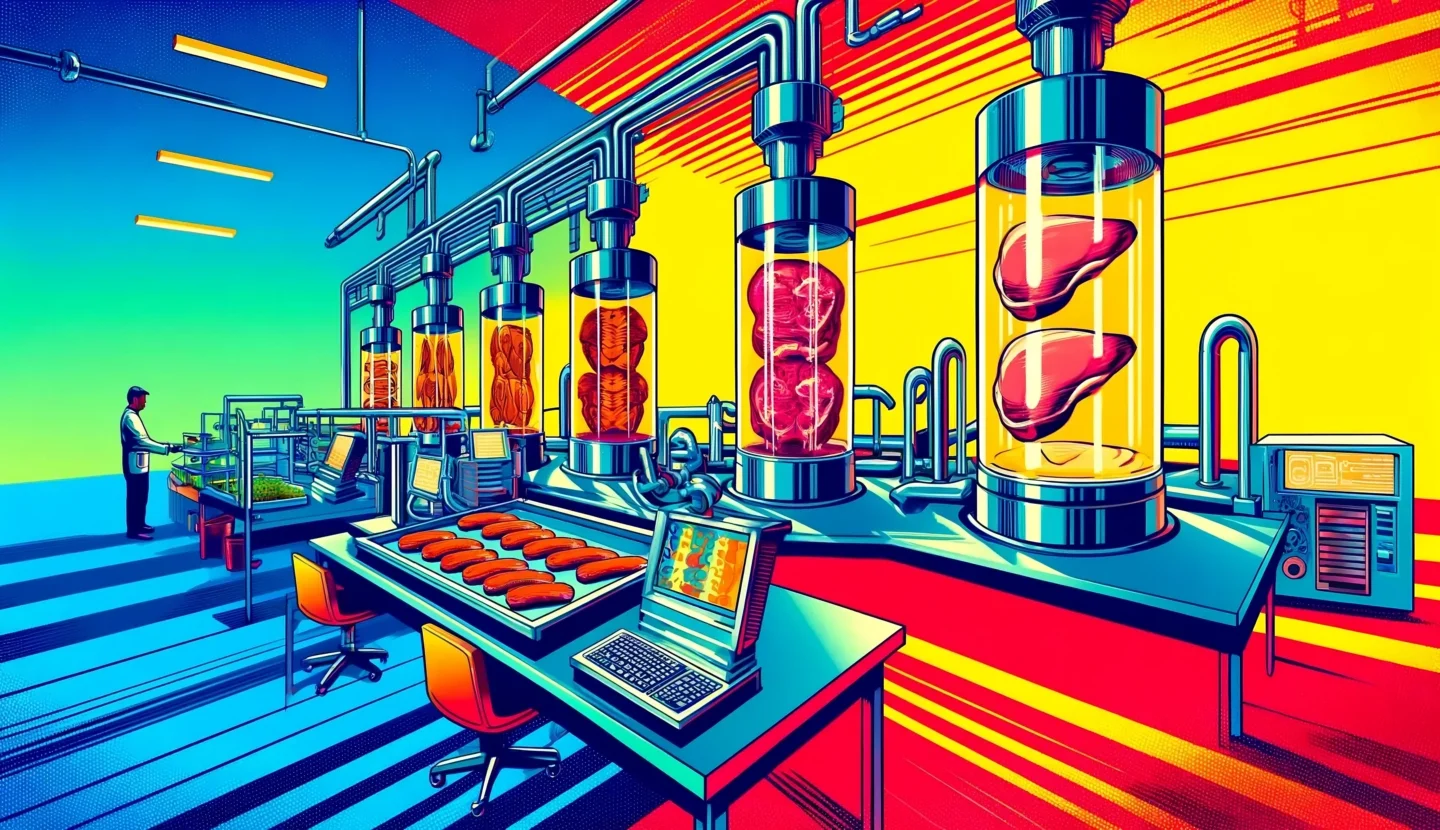
The constraint right now on factory farming is how far can you push the biology of these animals? But AI could remove that constraint. It could say, “Actually, we can push them further in these ways and these ways, and they still stay alive. And we’ve modelled out every possibility and we’ve found that it works.”
I think another possibility, which I don’t understand as well, is that AI could lock in current moral values. And I think in particular there’s a risk that if AI is learning from what we do as humans today, the lesson it’s going to learn is that it’s OK to tolerate mass cruelty, so long as it occurs behind closed doors. I think there’s a risk that if it learns that, then it perpetuates that value, and perhaps slows human moral progress on this issue.
Lewis Bollard
In today’s episode, host Luisa Rodriguez speaks to Lewis Bollard — director of the Farm Animal Welfare programme at Open Philanthropy — about the promising progress and future interventions to end the worst factory farming practices still around today.
They cover:
- The staggering scale of animal suffering in factory farms, and how it will only get worse without intervention.
- Work to improve farmed animal welfare that Open Philanthropy is excited about funding.
- The amazing recent progress made in farm animal welfare — including regulatory attention in the EU and a big win at the US Supreme Court — and the work that still needs to be done.
- The occasional tension between ending factory farming and curbing climate change.
- How AI could transform factory farming for better or worse — and Lewis’s fears that the technology will just help us maximise cruelty in the name of profit.
- How Lewis has updated his opinions or grantmaking as a result of new research on the “moral weights” of different species.
- Lewis’s personal journey working on farm animal welfare, and how he copes with the emotional toll of confronting the scale of animal suffering.
- How listeners can get involved in the growing movement to end factory farming — from career and volunteer opportunities to impactful donations.
- And much more.
Producer and editor: Keiran Harris
Audio engineering lead: Ben Cordell
Technical editing: Simon Monsour, Milo McGuire, and Dominic Armstrong
Additional content editing: Katy Moore and Luisa Rodriguez
Transcriptions: Katy Moore